Document Type
Article
Version
Publisher's PDF
Publication Title
Physical Review E
Volume
71
Publication Date
6-2005
Abstract
Given two time series X and Y, their mutual information, I(X, Y)= I(Y, X), is the average number of bits of X that can be predicted by measuring Y and vice versa. In the analysis of observational data, calculation of mutual information occurs in three contexts: identification of nonlinear correlation, determination of an optimal sampling interval, particularly when embedding data, and in the investigation of causal relationships with directed mutual information. In this contribution a minimum description length argument is used to determine the optimal number of elements to use when characterizing the distributions of X and Y. However, even when using partitions of the X and Y axis indicated by minimum description length, mutual information calculations performed with a uniform partition of the XY plane can give misleading results. This motivated the construction of an algorithm for calculating mutual information that uses an adaptive partition. This algorithm also incorporates an explicit test of the statistical independence of X and Y in a calculation that returns an assessment of the corresponding null hypothesis. The previously published Fraser-Swinney algorithm for calculating mutual information includes a sophisticated procedure for local adaptive control of the partitioning process. When the Fraser and Swinney algorithm and the algorithm constructed here are compared, they give very similar numerical results (less than 4% difference in a typical application). Detailed comparisons are possible when X and Y are correlated jointly Gaussian distributed because an analytic expression for I(X, Y) can be derived for that case. Based on these tests, three conclusions can be drawn. First, the algorithm constructed here has an advantage over the Fraser-Swinney algorithm in providing an explicit calculation of the probability of the null hypothesis that X and Y are independent. Second, the Fraser-Swinney algorithm is marginally the more accurate of the two algorithms when large data sets are used. With smaller data sets, however, the Fraser-Swinney algorithm reports structures that disappear when more data are available. Third, the algorithm constructed here requires about 0.5% of the computation time required by the Fraser-Swinney algorithm.
Publisher's Statement
© 2005 by the American Physical Society. The publisher's version of the article can be found at http://link.aps.org/doi/10.1103/PhysRevE.71.066208.
Citation
C.J. Cellucci, A.M. Albano and P.E. Rapp, Phys. Rev. E 71, 66208 (2005).
DOI
10.1103/PhysRevE.71.066208
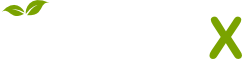
- Citations
- Citation Indexes: 158
- Usage
- Downloads: 3041
- Abstract Views: 113
- Captures
- Readers: 149
- Mentions
- Blog Mentions: 2